
Backlink data has been all the rage since the beginning of Google. On November 18th, 2011, Yahoo! shuttered its now defunct Site Explorer, but the SEO industry hardly missed a beat with tools like Moz’s Open Site Explorer and a huge wave of new entrants onto the scene.
As of 2015, there are a lot of industry writers out there pushing for more data: more links, fresher links, and more detailed link info than ever before.
So…just how much backlink data does one need? And, what do you do with it once you have it? The good news is that, today, there is a scientific way to predict, using a search engine model, exactly what the minimum set of backlink data will be for your next project.
It Matters How Links are Scored
Before I get into the details of how to use a search engine model to determine the minimum level of backlinks, we first must discuss what that backlink data needs to show in the first place.
If you aren’t familiar with PageRank, it’s algorithm determines the likelihood that a person randomly clicking on links will arrive at any particular page on the Internet. I won’t go into how it is calculated here, but think of this as a raw or gross ranking power metric for any given page on the Internet. In the screenshot below, it’s called Gross Total Link Flow (on Webpage).
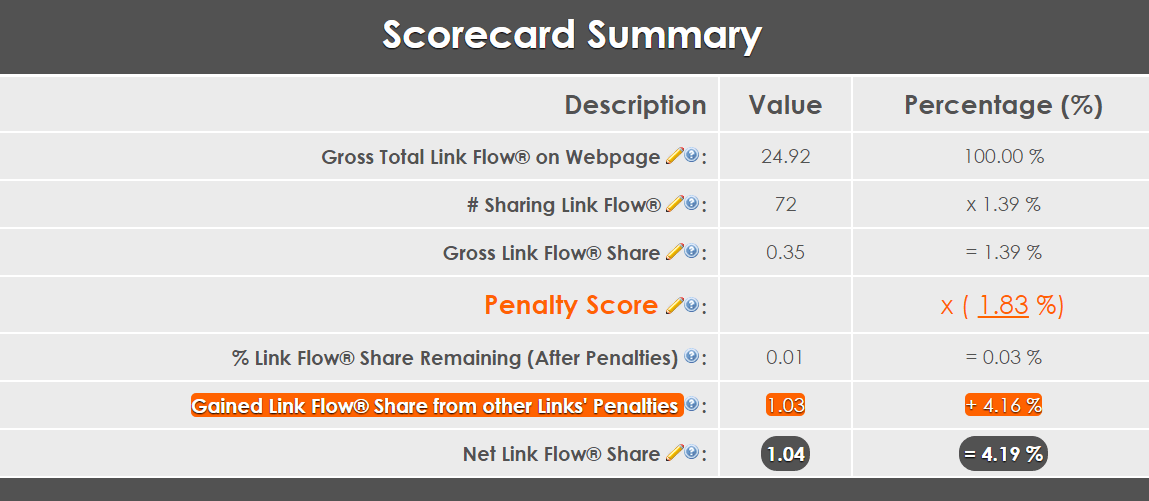
An example of how links are scored, with respect to one another.
In a modern search engine, each page’s link is scored with respect to one another. It is primarily a zero-sum game here: if one link is penalized, its loss is redistributed amongst the other links on that page. You start out with the raw ranking power, and it is then graded on a curve.
For example, if a link has a small font, that means it typically is getting a reduced link flow. However, if all the other links on that page are smaller in font, it actually gets a boost in link flow.
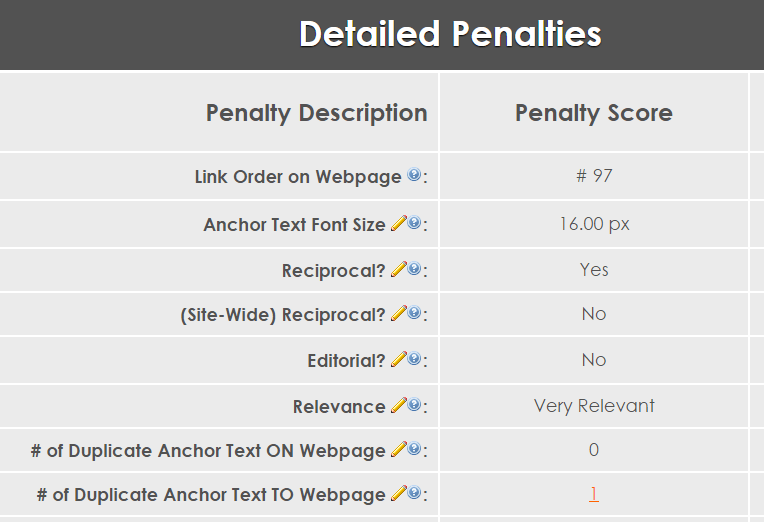
A sample list of link scoring algorithms.
Why am I talking about how links are scored? After all, we just want to know what the minimum backlink data is, not how it is scored.
Here’s why: take a look at some of the algorithms that are used when determining which links are spammy and which aren’t. How about a link relevance algorithm? How do we determine relevance without knowing all about the page that it is on and knowing all about the page that it is pointing to?
True Link Scoring, Relevance, and N-Order Scoring
The hard truth is that to determine how “relevant” a particular link is, we must do processing not only on the target page but also the source page. But to properly score the source page, we need its source scored as well, and so on…if it’s a popular page, we might have to crawl half of the Internet!
How can we simulate this link scoring with a finite (non-Mountain View) set of resources? You have two options here:
- Brute force it: buy a lot of compute time on Amazon, and build a very large search engine that will crawl (and score) each backlink, the backlinks of those backlinks, and so on, or
- Find a diminishing point of accuracy with regards to backlink data, reducing the data set to a sample size that can actually be used in a modern search engine model that calculates just like a search engine, but within a reasonable time frame.
Note that the first option has NOT been done by the backlink data provider of your choice. They have most certainly crawled most of the Internet, yes, and cataloged link data in a basic way. But, they are not properly simulating things to get true link metrics like link relevancy, neighborhood effects, and more. For that, it takes orders of magnitude more processing. Just ask any search engine startup in the past 15 years.
That leaves us with option two, which allows us to properly simulate link scoring just like the modern search engines do, but at the same time only having to dedicate a smaller, cost-effective set of resources to do it.
Option two will be the focus of the rest of this article, as I take you through the actual implementation of how you can establish this cutoff point of backlink data in a scientific and systematic way.
What Does a Typical Backlink Profile Look Like?
Before we get into how to implement option two, we first have to discuss what a typical backlink profile looks like, and how it affects a search engine. Why? Because we need to know which metric to use to sort all of the backlinks, assuring ourselves of the strongest subset of link data possible.
When I talk about sorting the “top” links in the backlink profile, am I talking about the ranking power of the page that the link is on? No. This metric, while widely popular within backlink data providers, is mostly useless in this type of statistical modeling problem. What we need to know is how much power each backlink is distributing to the target website.
In this example, we’ll call this ranking power “Link Flow Share” (in the screenshot above, it’s called “Net Link Flow Share”). If we sort by this Link Flow Share metric, we get a distribution that, for most backlink profiles, looks like this:
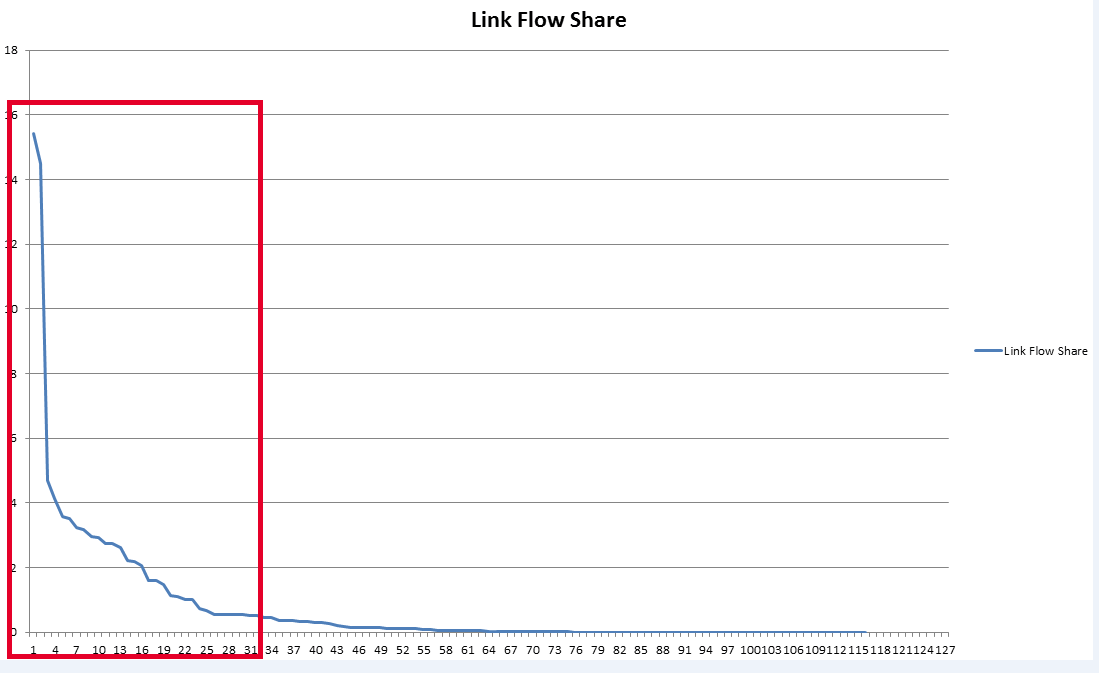
Typical Link Flow Share Distribution for Backlinks
What you will find is that the majority of this Link Flow Share is distributed from a top select group of links. While the “long tail” of Link Flow Share affects things like keyword positioning (for instance, a link’s anchor text actually influences the contextual meaning of its target page), the “head” is what affects the bulk of the Link Flow to that page.
Where is the Cutoff Point for the Sample Size?
Given that we can clearly see the majority of Link Flow Share resides within some “top % of pages” figure, we now have an ordered list of backlinks we can choose to truncate.
To do so, we first need to determine what the cutoff point would be for a given list of backlinks. That cutoff point should, at a minimum, not suffer from any loss of precision or accuracy when used in a statistical modeling environment. By definition, we can use our search engine model to measure the accuracy of a number of alternative subsets of backlink data.
In the screenshot below, you can see how a search engine model uses a similar “Net Total Link Flow Boost” figure to incorporate into its query scoring model.
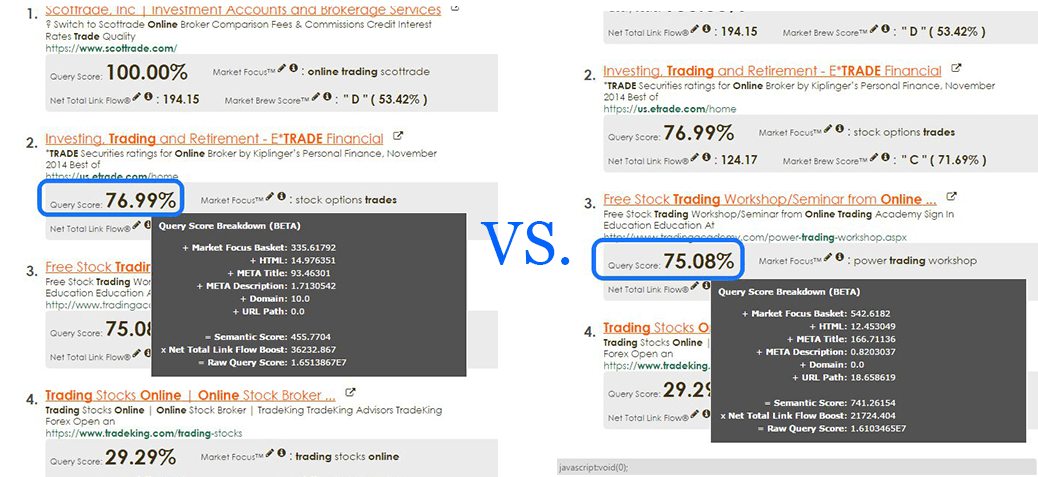
We can use a search engine model to determine the accuracy of each subset of backlinks
I have already written in great lengths about how to create a self-calibrated search engine model, which successfully curve-fits any given target search engine environment to a statistical model. When the process is completed, we can easily measure how close this model is versus the actual search results.
Using this process allows us to quickly determine “how much backlink data” we need. It goes a little like this:
- Start with the top 10% of backlinks for a group of websites, and run that into our search engine model. Determine how well the model self-calibrates itself to reality.
- Next, reduce that backlink sample to 5% of the total backlinks, again determining how well the search engine model self-calibrates itself to reality.
- If the accuracy of the model drops by a minimum threshold percentage, then stop. You have found your cutoff point. If not, go back to step 2 and drop the subset of backlink data to 3%, 2%, 1% and so on, until you have found your cutoff point.
Each time we will have the search engine model self-calibrate itself to the target search engine environment, and each time we will measure how well the self-calibration did.
If the model cannot converge on a high correlation of the real world results, then we know we’ve sampled too little. And once we see that the increased correlation is insignificant, we know we’ve sampled too much.
Conclusion
Having backlink data is great, but more is not always better. Using a search engine model, I showed you how to determine the cutoff point of backlink data needed for any project.
You also learned that it’s the proper scoring of Link Flow Share that counts more than just knowing how powerful the page that the link is on. You learned that quality (via real-world link scoring), not quantity, of backlink data makes all the difference in the quality of your results.
Most importantly, you can now arrive at very accurate modeling of a given search engine environment, without having to build your own massive search engine.
No comments:
Post a Comment